Neural Surface Detection for Unsigned Distance Fields
Paper accepted to ECCV 2024
Federico Stella, Nicolas Talabot, Hieu Le, Pascal Fua
Feel free to contact us for any questions!
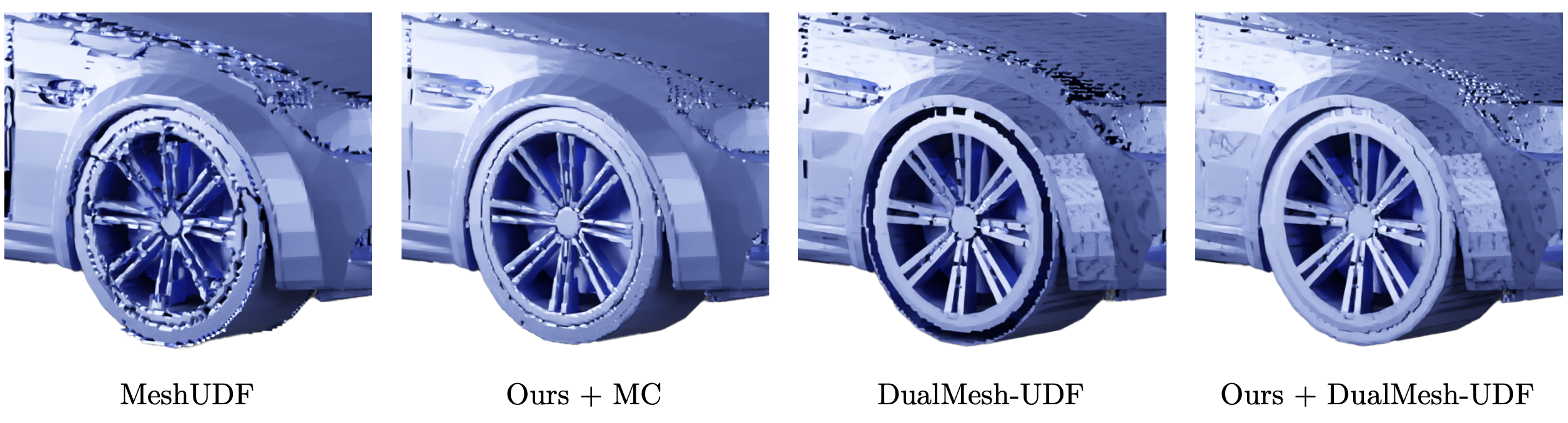
Abstract
Extracting surfaces from Signed Distance Fields (SDFs) can be accomplished using traditional algorithms, such as Marching Cubes. However, since they rely on sign flips across the surface, these algorithms cannot be used directly on Unsigned Distance Fields (UDFs). In this work, we introduce a deep-learning approach to taking a UDF and turning it locally into an SDF, so that it can be effectively triangulated using existing algorithms. We show that it achieves better accuracy in surface detection than existing methods. Furthermore it generalizes well to unseen shapes and datasets, while being parallelizable. We also demonstrate the flexibily of the method by using it in conjunction with DualMeshUDF, a state of the art dual meshing method that can operate on UDFs, improving its results and removing the need to tune its parameters.Video explanation
Pipeline
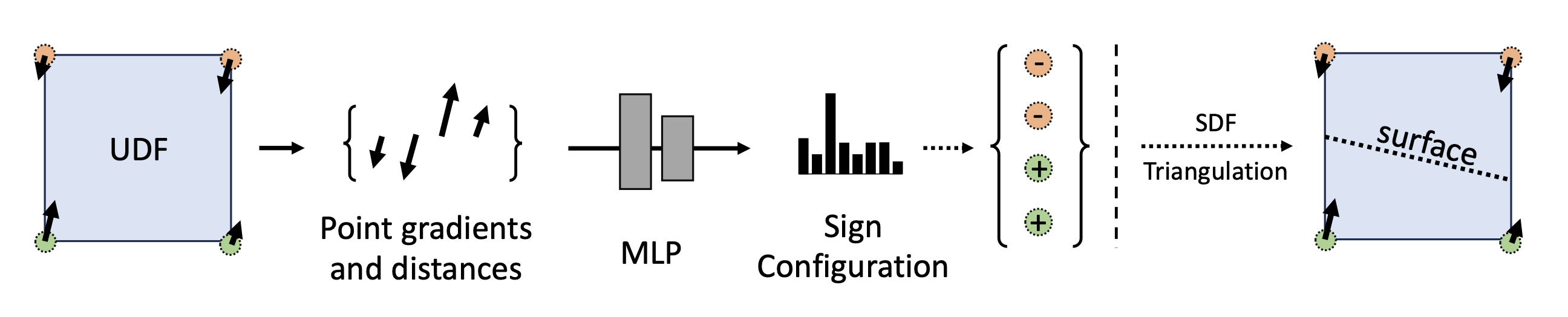
We formulate the surface detection problem as a per-cell classification task. In each cell, we map point distances and gradients to a sign configuration of the cell vertices, which can be used to mesh the surface via Marching Cubes or Dual Contouring.
Quantitative Evaluations
We compute Chamfer Distance and Image Consistency over hundreds of shapes to find the following results.
Note: DCUDF can achieve better accuracy by removing the cutting step. However this would make the surface double layered.
Note: DCUDF can achieve better accuracy by removing the cutting step. However this would make the surface double layered.
MC-based methods
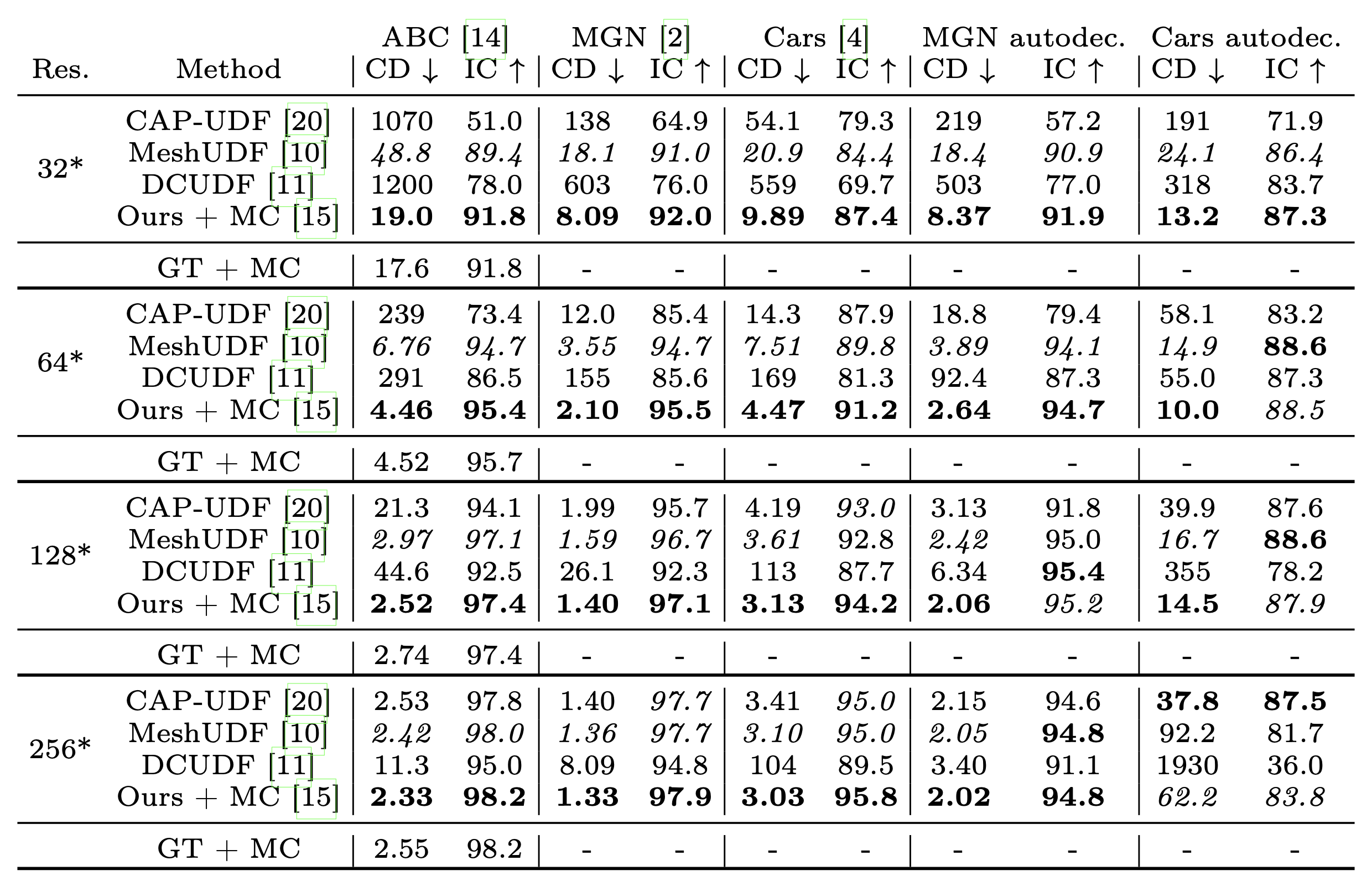
DC-based methods
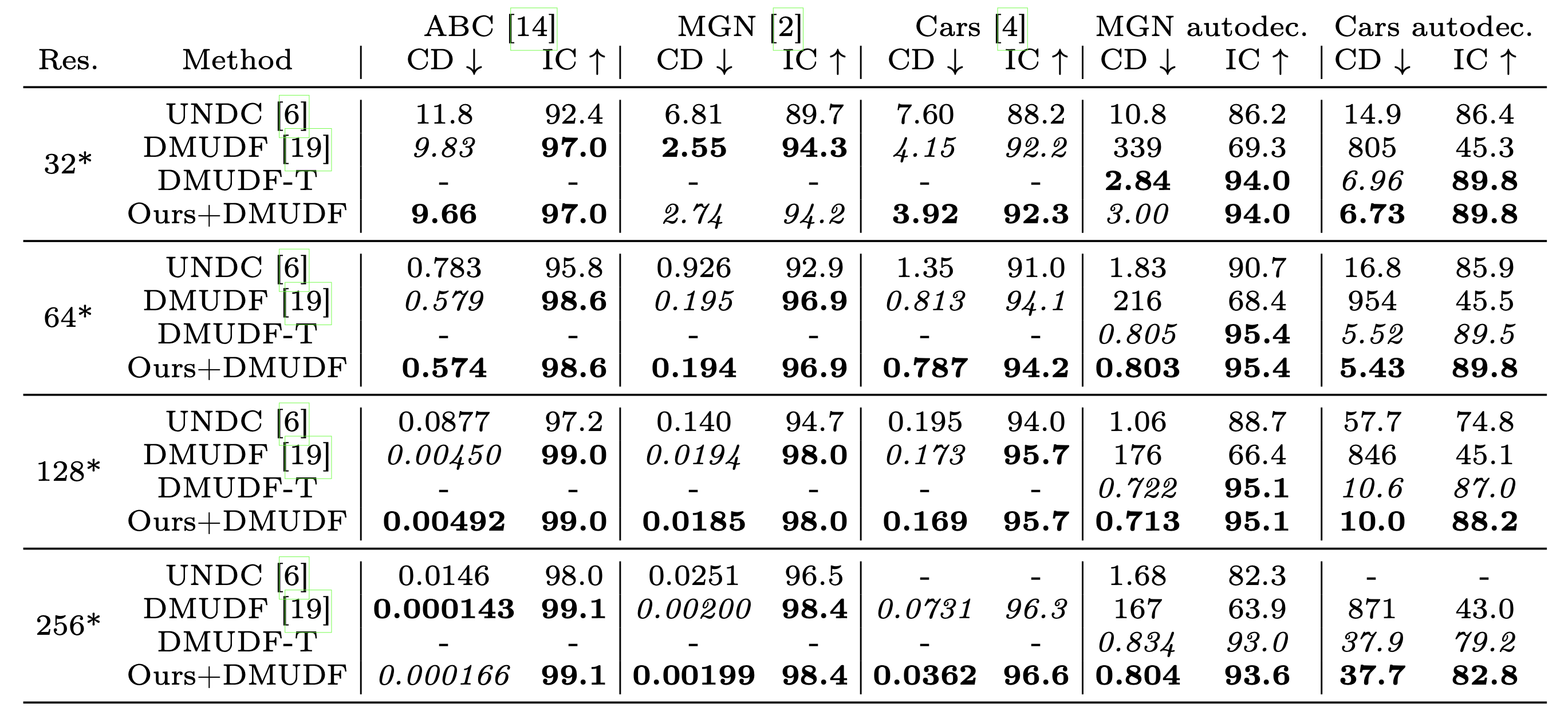
BibTeX
If you find our work useful, please cite it:
@InProceedings{stella2024nsdudf,
author="Stella, Federico and Talabot, Nicolas and Le, Hieu and Fua, Pascal",
title="Neural Surface Detection for Unsigned Distance Fields",
booktitle="European Conference on Computer Vision",
year="2024",
publisher="Springer",
pages="394--409",
isbn="978-3-031-73636-0"
}